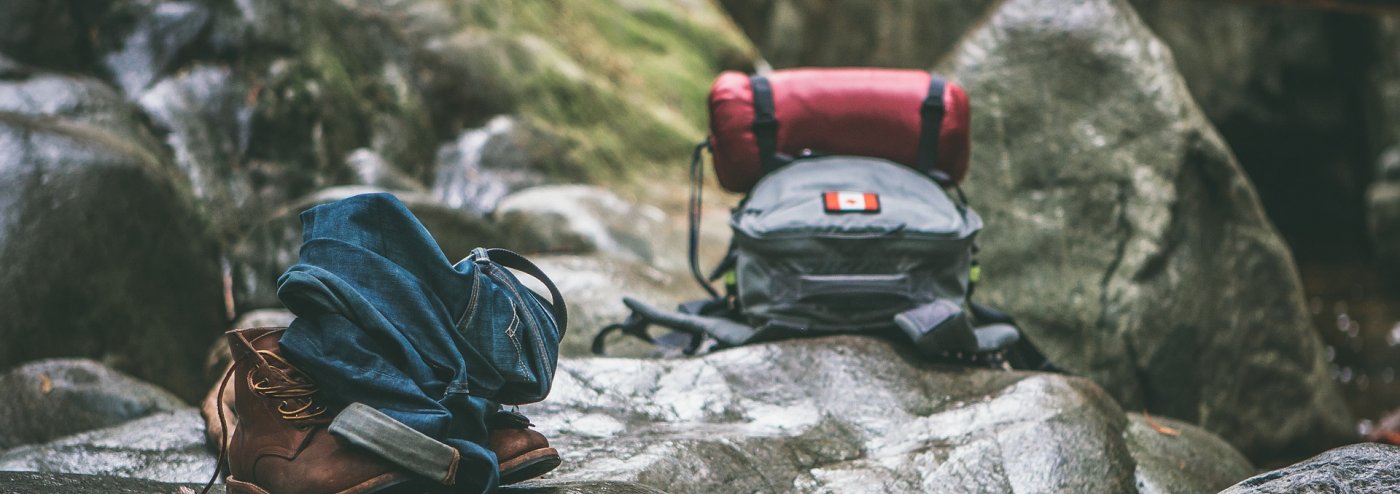
Computer vision to identify recreational activities in social media images
Our first paper on quantifying cultural ecosystem services using Computer Vision is published in People and Nature (https://besjournals.onlinelibrary.wiley.com/doi/10.1002/pan3.10382). The study was jointly carried out by the Outdoor R&D group of the University of Washington in Seattle (https://www.outdoorrd.org) and our group members Heera Lee and Bumsuk Seo.
Plain language summary from the Journal blog:
recCNNize – an AI tool to identify recreational activities in social media images
Visitors hiking in the Cascade Mountains, Washington, USA with white boxes indicating parts of
the image that the AI tool might identify as important to the classification. White text is an
example of model output, showing the probability that the image belongs to each class.
(Photo by Sama Winder, annotations added for demonstration).
People share a lot of information on social media. This includes images of where they have different types of outdoor recreation experiences. Sorting through the millions of images that people share on social media is not feasible, however, so we created a computer vision tool called recCNNize that uses artificial intelligence to identify images of recreational activities such as hiking, biking, and birding. Our study introduces, tests, and applies this tool to more than 30,000 photographs taken in a national forest in Washington, USA. This research is unique in a few key ways.
First, our tool is entirely open source, and we are sharing it publicly so that others can use and improve it. Most prior research uses proprietary algorithms that are created by for-profit companies who frequently make undocumented changes to their tools and who is allowed to use them. Our paper discusses the strengths and weaknesses of our tool so others can make informed decisions about how to interpret our results or apply the tool themselves.
Second, our study quantifies biases in what is represented in people’s social media feeds by comparing our results to an on-site survey of visitors to the same destinations. We find that there are major differences between the activities identified in social media and the activities that visitors self-report on a survey. This highlights one of the pitfalls of research that relies on social media – we can not assume that social media and social media users are representative of everyone who recreates outdoors.
Finally, we use our new method to create maps of the number of distinct activities that visitors engage in and post about across two regions in Washington, USA. Using this map, we find that images on social media show a greater number of activities in places that have trails, are easily accessible from a road, and include access to rivers or lakes. Results like these help managers decide how to improve people’s recreational experiences. In Washington, for example, National Forests might consider building or improving trails that lead to areas with rivers and lakes in order to support a broader number of outdoor activities.
Additional information
Original article: https://besjournals.onlinelibrary.wiley.com/doi/10.1002/pan3.10382
DOI: 10.1002/pan3.10382
Abstract:
Environmental management increasingly relies on information about ecosystem services for decision-making. Compared with regulating and provisioning services, cultural ecosystem services (CES) are particularly challenging to characterize and measure at management-relevant spatial scales, which has hindered their consideration in practice. Social media are one source of spatially explicit data on where environments support various types of CES, including physical activity. As tools for automating social media content analysis with artificial intelligence (AI) become more commonplace, studies are promoting the potential for AI and social media to provide new insights into CES. Few studies, however, have evaluated what biases are inherent to this approach and whether it is truly reproducible.
This study introduces and applies a novel and open-source convolutional neural network model that uses computer vision to recognize recreational activities in the content of photographs shared as social media. We train a model to recognize 12 common recreational activities to map one aspect of recreation in a national forest in Washington, USA, based on images uploaded to Flickr. The image classifier performs well, overall, but varies by activity type. The model, which is trained with data from one region, performs nearly as well in a novel region of the same national forest, suggesting that it is broadly applicable across similar public lands. By comparing the results from our CNN model with an on-site survey, we find that there are apparent biases in which activities visitors choose to photograph and post to social media. After considering potential issues with underlying data and models, we map activity diversity and find that natural features (such as rivers, lakes and higher elevations) and some built infrastructure (campgrounds, trails, roads) support a greater diversity of activities in this region.
We make our model and training weights available in open-source software, to facilitate reproducibility and further model development by researchers who seek to understand recreational values at management-relevant scales—and more broadly provide an example of how to build, test and apply AI to understand recreation and other types of CESs.